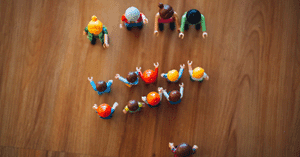
Improper uses of ML
Machine learning in its native form is only as good as the data it learns from. ML can suffer from equity gaps when the underlying data is sampled inadequately to represent population heterogeneity. There is plenty of evidence that clinical trials lack racial and ethnic diversity, thus requiring pharmacovigilance and prompting the FDA to endorse the concept of real-world data and real-world evidence. Another example is the failure of EPIC’s sepsis predictive algorithm in a recent Michigan trial. This potential problem is particularly acute for underrepresented groups if there are large gaps in model targets between groups and phenotypic or fixed variables that separate these groups are used in machine learning.
On a related note, a prevalent yet avoidable equity-gap issue in machine learning is over-reliance on non-malleable, phenotypic, easily-observable, group-level variables instead of investing in deriving malleable, influenceable hidden variables at an individual level. Demographic variables fall into the former category. Such a practice can be deadly if model scores are used to determine school/program admission, scholarship, loan approval, treatment, etc. While there are legal protections, subtle biases in models that use such simple variables can exacerbate equity gaps.
Another well-known shortcoming of risk-prediction models is that improper use of dependent variables can lead to unintended biases. For example, population health management risk models typically predict year-2 costs based on year-1 health data. Using such risk models can exacerbate equity gaps because patients who have unequal access to healthcare tend to have lower costs while the underlying drivers of sickness for such patients can be greater. As a result, those with unequal healthcare access can have even less care despite having potentially greater sickness drivers since risk models will prioritize those with higher predicted costs.
The same can be said about predicting student persistence. Too much focus on short-term metrics in machine learning can lead to the ignorance of festering and insidious risk factors that can hurt long-term student success, such as completion and job success post graduation. Furthermore, too much of advising resources can be put into improving short-term KPIs, such as (1) advising students to switch to “easier” majors while overlooking students who are off pathways but persist, (2) nudging students to register early without sufficient planning in course offering/schedule optimization, and (3) lowering standards in gatekeeper courses that may postpone the inevitable down the road.
Another major potential failure in machine learning can happen due to recency bias. Models trained using the most recent data can forget what can happen during different time periods where data relationships can change significantly. This factor was one of the major reasons for Zillow’s house flipping model failure leading to significant layoffs and mispricing of risks in mortgage-backed securities (MBS) during the 2008 Great Recession contributing to major setback in economic gains of the underprivileged and hurting those with lower socioeconomic status particularly hard. The MBS pricing error occurred because the pricing models were based on the most recent 3 years of data, during which home prices kept rising.
Finally, there can be too much resource allocation into improving model performance instead of taking a balanced approach to translating machine learning outputs with the goal of taking actions on prescriptive insights that can lead to improved success outcomes. There are calls for maximizing clinical utility, not predictive accuracy, in clinical settings to improve patient care. Trying to maximize predictive accuracy through the use of highly sophisticated models can backfire when there are significant differences between training and operational data, especially with insufficient MLOps to guard for data nonstationarity.
Our approach to lower equity gaps and maximize human-AI synergy
The more decision makers know of these potential failure points in machine learning, the greater the probability of such failures being prevented. There are four major approaches that can accommodate the aforementioned model failure points and bring out much-needed synergy between humans and AI/ML systems in order to help lower equity gaps.
Feature engineering and crowdsourcing
The first and most effective machine learning approach to deal with the improper use cases on ML is to invest in time-series feature engineering and crowdsource analytics to make models more transparent, easily explainable, and actionable. What matters most from an actionability perspective is the underlying, hidden psycho-social behavioral factors that can be inferred through judicious feature engineering of time-series event data and ecological momentary assessment. For instance, features derived from micro surveys, social networks, and activity event data were leveraged to quantify the effects of social and motivational factors crucial in spreading good health behaviors through one’s social network.
Crowdsourcing facilitates learning from those who have been through similar experiences. Crowdsourcing combined with human capital analytics is particularly effective in democratizing knowledge from the best experts from the perspective of enterprise knowledge retention. Similarly, from data, characteristics of students who overcome adversities to do well can be mined and disseminated to students who are currently experiencing similar adversities through crowdsourcing analytics. Another example is to analyze communication patterns of instructors in higher education or primary care physicians who improve their student engagement and patient activation levels, respectively. Figure 1 shows an example of crowdsourcing in healthcare from CureTogether, highlighting treatments for depression ranked by effectiveness and popularity.
Figure 1: Crowdsourced knowledge on effective treatments for depression from CureTogether.
Using more than one success metric
It is much more effective to predict surrogate variables that are faster and leading indicators for future adverse health events, such as HbA1c and patient activation level for diabetics , instead of just focusing on healthcare costs. Having a representative set of outcomes or dependent variables brings a balanced view of likely future states so interventions can be designed for the best overall success outcome as opposed to short-term, narrowly-focused KPIs. For example, increasing Rx costs for improved medication adherence is beneficial if Rx cost increase is accompanied by drops in HbA1c, ER visits, and inpatient episodes.
With that said, it should be abundantly clear that there must be strong associations between short-term KPIs and long-term success metrics. For example, improving a student’s soft skills that employers desire is bound to improve their career success. Similarly, lowering a patient’s HbA1c can lead to long-term reduction in healthcare costs despite short-term increase in medication costs, especially when HbA1c reduction is accompanied by increase in patient activation.
Moving beyond predictions to bring out the best in human creativity
Machine learning models cannot be a black box. Leveraging the first two approaches, predictive models can be humanized to gain trust and to encourage action from their users. Risk scores by themselves do not lead to equity-gap reduction. In order to facilitate action, prescriptive machine learning is of paramount importance.
In order to recommend prescribed actions, there needs to be evidence of effectiveness. This is the core of causal ML. Causal ML is the bridge between real-world evidence (RWE) collected from retrospective data and prospective experiments of promising interventions based on evidence. This is where human creativity can come into play, helping student success teams do their best work, by evaluating evidence, identifying areas of improvement based on causal insights, and catalyzing process innovation that can be sustained.
MLOps to account for heterogeneities in data and people MLOps is where all these nuanced approaches can be weaved in so that dynamic AI/ML systems can be built in a sustainable manner. Data and target nonstationarities are tracked continuously, resulting in model retrain using appropriate training data. Furthermore, a succinct, yet comprehensive output is generated for human consumption and decision making.
Summary and future directions
As machine learning models become more commoditized, more emphasis will be placed on connecting risk model outputs to success outcomes. Lately, machine-learning vulnerabilities have been in full display with self-driving cars, self-learning chatbots, and ML models applied to quick-profit opportunities, where failures lead to human costs and higher levels of inequities given human costs are not equally distributed. What’s sorely needed is a balanced approach to all kinds of machine learning analytics with attention to detail, which gets to the core of hidden variables that explain why we do what we do, and how we can reshape trajectories in a more favorable direction to help lower equity gaps, improve success rates of equitable interventions, and learn from causal ML insights.